The rat in a maze may be one of the most classic research motifs in brain science, but together with international collaborators we describe a new innovation in Cell Reports that shows just how far such experiments are still pushing the cutting edge of technology and neuroscience.
In recent years, scientists have shown that by recording the electrical activity of groups of neurons in key areas of the brain, they could read a rat’s thoughts of where it was, both after it actually ran the maze and also later when it would dream of running the maze in its sleep—a key process in memory consolidation.
In a new study, several of the scientists involved in pioneering such “mind-reading” methods now report they can read out those signals in real-time as the rat runs the maze, with a high degree of accuracy.
A chip for real-time recording
The findings are the result of a team effort by researchers at NYU, MIT and NERF. The team showed that by implementing their neural decoding software on a graphical processing unit (GPU) chip—the same kind of highly parallel processing hardware favored by video gamers—they were able to achieve an unprecedented increase in decoding and analysis speed.
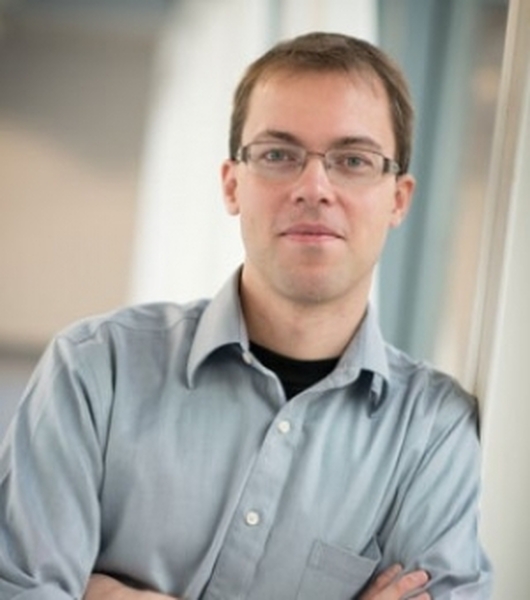
“Our GPU-based system is 20 to 50 times faster than conventional multi-core CPU chips. We also show that the system remains rapid and accurate even when handling a simulation of more than a thousand input channels.”
The scientists also report the ability for the software to provide a rapid statistical assessment of whether a set of reactivated neural spatiotemporal activity patterns truly pertains to the task, or is perhaps unrelated.
“We propose an elegant solution using GPU computing to not only decode information on the fly but also to evaluate the significance of the information on the fly,” said Zhe Chen, professor at NYU and co-senior author on the paper.
Faster than ever
Prior experiments recording neural representations of place have helped to show that animals replay their spatial experiences during sleep and have allowed researchers to understand more about how animals rely on memory when making decisions about navigation. Traditionally, though, the brain readings have been analyzed after the fact, or “offline.”
More recently, scientists have begun to perform real-time analyses but these have been limited both in detail and reliability. The team of Fabian Kloosterman published a paper in eLife just last month, on a real-time, closed-loop read-out of hippocampal neurons as rats navigated a 3-arm maze. That system used multi-core CPUs.
“We now demonstrate a faster GPU-based implementation of our real-time neural decoding algorithm so that it scales to data from larger neural probes, for example the neuropixels probe,” says Kloosterman. The software of the system is freely available and open source.
“We predict these experiments will produce new insights into how these replay events drive memory formation and behavior.”
Closing the loop
The new GPU system will bring the field even closer to having a detailed, real-time and highly scalable understanding of the content of a rat’s thoughts. By combining these capabilities with optogenetics – a technology that makes neurons controllable with flashes of light – the researchers could conduct “closed-loop” studies in which they use their instantaneous readout of spatial thinking to trigger experimental manipulations. For example, they could see what happens to navigational performance the day after interfering with replay during sleep, or what temporarily disrupting communication between the cortex and hippocampus might do when a rat faces a key decision about which direction to go.
“The ability to so robustly track the rat’s spatial representations in real-time opens the door to a whole new class of experiments,” says Chen. “We predict these experiments will produce new insights into how these replay events drive memory formation and behavior.”
Read more
Real-Time Readout of Large-Scale Unsorted Neural Ensemble Place Codes
Hu et al (2018) Cell Reports 25(10):2635-2642.e5